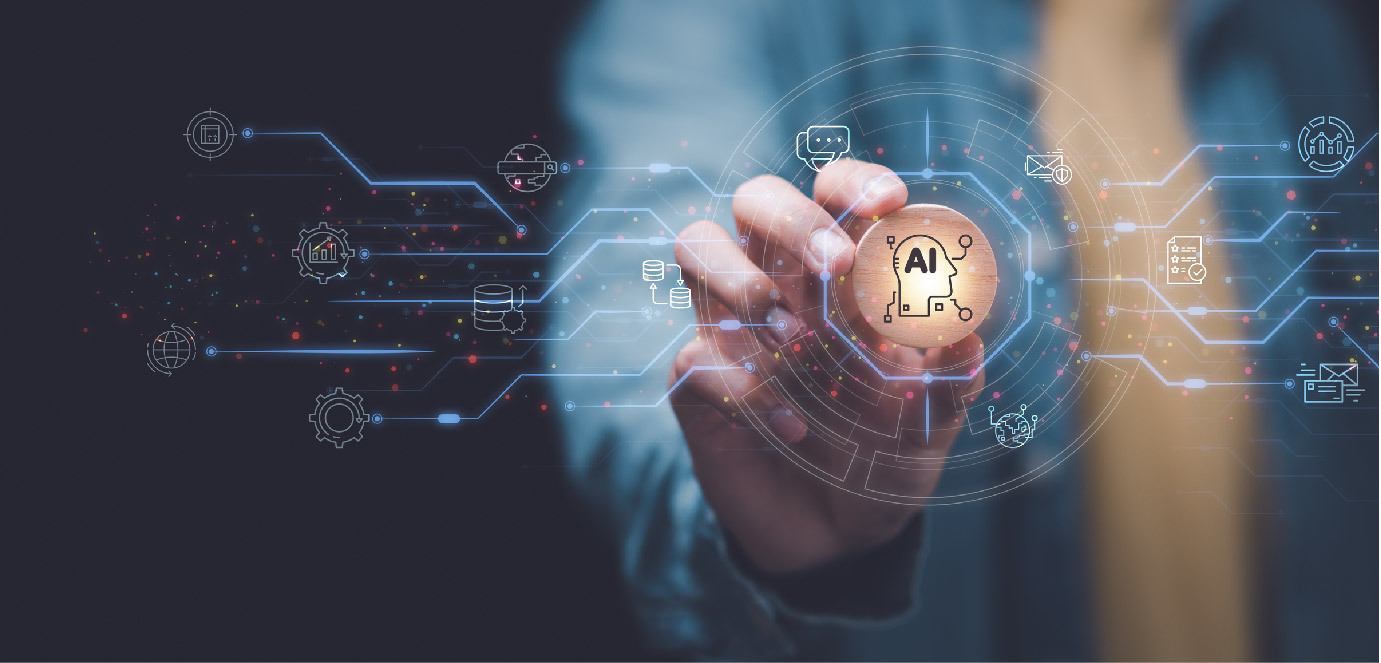
MIT Researchers Develop Comprehensive AI Risk Repository: A Living Database to Tackle AI Dangers
Artificial intelligence (AI) holds immense potential, but it also brings a wide array of risks. Researchers at the Massachusetts Institute of Technology (MIT) are taking a proactive step by not only identifying these risks but also creating what they describe as “a living database” to track and manage them. This initiative, led by MIT’s Computer Science & Artificial Intelligence Laboratory (CSAIL) and the FutureTech Group, aims to offer a clearer understanding of AI’s risks through the AI Risk Repository.
According to MIT Technology Review, adopting AI is not without dangers. From biased systems to the spread of misinformation, AI technologies have a myriad of potential issues. To manage these risks effectively, it’s crucial first to understand and categorize them, which is where MIT’s initiative steps in.
The AI Risk Repository was developed to address gaps in understanding and managing AI risks. MIT researchers discovered that no single framework could capture all the risks posed by AI. In fact, their analysis found that even the most comprehensive AI risk frameworks miss around 30% of potential risks.
Dr. Peter Slattery, the lead researcher, emphasized the fragmented nature of the AI risk literature. He raised concerns that policymakers and decision-makers might inadvertently overlook critical risks if they rely on incomplete information. The goal of the Repository is to present a comprehensive and regularly updated database that brings together all these scattered sources.
According to MIT, the Repository is built from a thorough review of 43 different AI risk frameworks and taxonomies, resulting in over 777 identified risks. These are continuously updated to provide a real-time reference for researchers, developers, policymakers, and other stakeholders.
The Repository consists of three core components:
• AI Risk Database: A collection of over 700 AI risks derived from the 43 frameworks, complete with quotes and source details.
• Causal Taxonomy of AI Risks: A classification system that explains when, how, and why each risk occurs.
• Domain Taxonomy of AI Risks: A system that categorizes risks into seven key domains and 23 subdomains, such as discrimination, privacy breaches, misinformation, and AI system failures.
These elements provide a structured view of the dangers posed by AI, offering a practical tool for anyone involved in AI development or governance.
Despite its benefits, MIT acknowledges that the Repository has limitations. It is based only on the 43 frameworks it reviewed, so it may miss emerging or unpublished risks. Additionally, the system relies on a single expert for coding and categorization, which introduces the possibility of bias or error. Still, the initiative’s potential impact is significant. Neil Thompson, director of MIT FutureTech and a key figure behind the Repository, noted that the database demonstrates the vast range of AI risks, many of which cannot be predicted in advance.
MIT’s AI Risk Repository is a first-of-its-kind effort to systematically gather, analyze, and share AI risk information. It provides a foundation for a more coordinated approach to addressing AI risks, with an emphasis on shared knowledge and continuous updates. As AI technology advances rapidly, the work of the MIT team represents a crucial step toward managing the risks that come with it. Their database offers a framework for responsible AI use, providing a more thoughtful, measured approach to AI deployment and its impact on society.